A post by Henry Bourne, PhD student on the Compass programme.
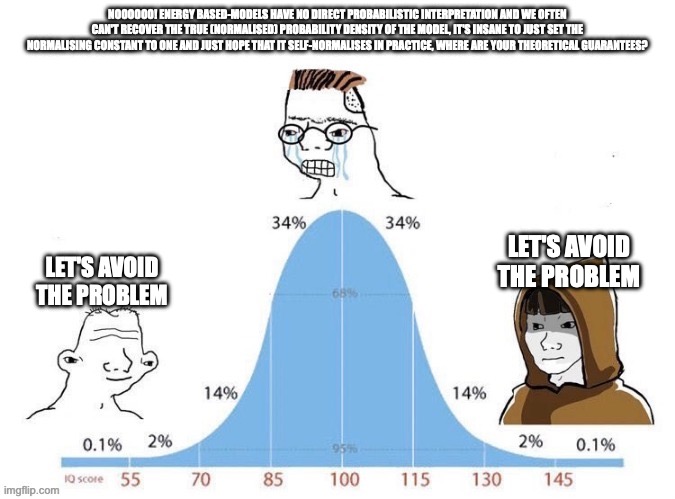
Currently I’ve been researching Noise Contrastive Estimation (NCE) techniques for representation learning aided by my supervisor Dr. Rihuan Ke. Representation learning concerns itself with learning low-dimensional representations of high-dimensional data that can then be used to quickly solve a general downstream task, eg. after learning general representations for images you could quickly and cheaply train a classification model on top of the representations.
NCE is a general estimator for parametrised probability models as I will explain in this blogpost. However, it can also be cleverly used to learn useful representations in an unsupervised (or equivalently self-supervised) manner, which I will also explain. I’ll start by explaining the problem that NCE was created to solve, then provide a quick comparison to other methods, explain how researchers have built on this method to carry out representation learning and finally discuss what I am currently working on.
NCE solves the problem of computing a normalising constant by avoiding the problem altogether and solving some other proxy problem. Methods that are able to model unnormalised probability models are known as Energy Based Models (EBM’s). We will begin by describing the problem with the normalising constant before getting on to how we will avoid it.
The problem … with the normalising constant
Let’s say we have some arbitrary probability distribution, $p_{d}(\cdot)$, and a parametrised probability model, $p_{m}(\cdot ; \alpha)$, which we would like to accurately model the underlying probability distribution. Let’s further assume that we’ve picked our model well such that $\exists \alpha^{*}$ such that $p_{d}(\cdot) = p_{m}(\cdot ; \alpha^{*})$.
Let’s just fit it to our data sampled from the underlying distribution using Maximum Likelihood Estimation! Sounds like a good idea, MLE has been extensively used, is reliable, is efficient and achieves the Cramer-Rao lower bound (the lowest possible bound an unbiased estimator can achieve for its variance/MSE), is asymptotically normal, is consistent, is unbiased and doesn’t assume normality. Moreover, there are a lot of tweaked MLE techniques out there that you can use if you would like an estimator with slightly different properties.
First let’s look under the hood of our probability model, we can write it as so:
$
\begin{array}{l|l}
p_{m}(\cdot;\alpha)=\frac{p_{m}^{0}(\cdot; \alpha)}{Z(\alpha)} & \text{where,} \: Z(\alpha) = \int p_{m}^{0}(u; \alpha) du
\end{array}
$
The likelihood is our probability model for some $\alpha$ evaluated over our dataset. Evaluating the likelihood becomes tricky when there isn’t an analytical solution for the normalisation term, $Z(\alpha)$, and the possible set of values $u$ can take becomes large. For example if we would like to learn a probability distribution over images then this normalisation term becomes intractable.
By working with the log we get better numerical stability, it makes things easier to read and it makes calculations and taking derivatives easier. So, let’s take the log of the above:
$
\begin{aligned}
&{} p_{m}(\cdot;\alpha) = \frac{p_{m}^{0}(\cdot; \alpha)}{Z(\alpha)} \\
& \Rightarrow \log p_{m}(\cdot; \theta) = \log p_{m}^{0} (\cdot ; \alpha) +c
\end{aligned}
$ |
$\text{Where, } \\ \theta = \{\alpha, c \}, \\ \text{c an estimate of} -\log Z(\alpha)$ |
Where, we write $p_{m}^{0}(\cdot;\alpha)$ to represent our unnormalized probability model. After taking the $\log$ we can write our normalising constant as $c$ and then include it as a parameter of our model. So, our new model now parameterised by $\theta$, $p_{m}(\cdot;\theta)$, is self-normalising, ie. it estimates it’s normalising constant. Another approach to make the model self-normalising would be to simply set $c=0$, implicitly making the model self-normalising. This is what is normally done in practice, but it assumes that your model is complex enough to be able to indirectly model $c$.
Couldn’t we just use MLE to estimate $\log p_{m}(\cdot ; \theta)$? No we can’t! This is because the likelihood can be made arbitrarily large by making $c$ large.
This is where Noise Contrastive Estimation (NCE) comes in. NCE has been shown theoretically and empirically to be a good estimator when taking this self-normalizing assumption. We’ll assess it versus competing methods at the end of the blogpost. But before we do that let’s first describe the original NCE method named binary-NCE [1] later we will mention some of the more complex versions of this estimator.
Binary-NCE
The idea with binary-NCE [1] is that by avoiding our problems we fix our problems! ie. We would like to create and solve an ‘easier’ proxy problem which in the process solves our original problem.
Let’s say we have some noise-distribution, $p_{n}(\cdot)$, which is easy to sample from, allows for an analytical expression of $\log p_{n} (\cdot)$ and is in some way similar to our $p_{d}(\cdot)$ (our underlying probability distribution which we are trying to estimate). We would also like $p_{n}(\cdot)$ to be non-zero wherever $p_{d}(\cdot)$ is non-zero. Don’t worry too much about these assumptions as they are normally quite easy to satisfy, apart from an analytical expression being available. They just are necessary for our theoretical properties to hold and for binary-NCE to work in practice.
We would like to create and solve a proxy problem where given a sample we would like to classify whether it was drawn from our probability model or from our noise distribution. Consider the following density ratio.
$
\begin{aligned}
\frac{p_{m}(u;\alpha)}{p_{n}(u)}
\end{aligned}
$
If this density ratio is bigger than one then it means that $u$ is more likely to have come from our probability model, $p_{m}(\cdot;\alpha)$. If it is smaller than one then $u$ is more likely to have come from our noise distribution, $p_{n}(\cdot)$. Therefore, if we can model this density ratio then we will have a model for how likely a sample is to have come from our probability model as opposed to have being sampled from our noise distribution.
Notice that we are modelling our normalised probability model above, we can rewrite it in terms of our unnormalised probability model as follows.
$
\begin{aligned}
& \log \left(\frac{p_{m}(u;\alpha)}{p_{n}(u)} \right) \\
& = \log \left(\frac{p_{m}^{0}(u;\alpha)}{Z(\alpha)} \cdot \frac{1}{p_{n}(u)} \right) \\
& = \log \left(\frac{p_{m}^{0}(u;\alpha)}{p_{n}(u)} \right) +c \\
& = \log p_{m}^{0}(u;\alpha) + c – \log p_{n}(u) \\
& = \log p_{m}(u;\theta) – \log p_{n}(u)
\end{aligned}
$
Let’s now define a score function $s$ that we will use to model our rewrite of the density ratio just above:
$
\begin{aligned}
s(u;\theta) = \log p_{m}(u;\theta) – log p_{n}(u)
\end{aligned}
$
One further step before introducing our objective function. We would like to model our score function somewhat as a probability, we would also like our model to not just increase the score indefinitely. So we will put our modelled density ratio through the sigmoid/ logistic function.
$
\begin{aligned}
\sigma(s(u;\theta)) = \frac{1}{1+ \exp(-s(u;\theta))}
\end{aligned}
$
We would like to classify according to our model of the density ratio whether the sample is ‘real’ / ‘positive or just ‘noise’/ ‘fake’/ ‘negative’. So a natural choice for the objective function is the cross-entropy loss.
$
\begin{aligned}
J(\theta) = \frac{1}{2N} \sum_{n} \log [ \sigma(s(x_{n};\theta))] + \log [1- \sigma(s(x_{n}’;\theta))]
\end{aligned}
$
Where $x_{i} \sim p_{d}$, $x_{i}’ \sim p_{n}$ for $i \in \{1,…,N\}$. Here we simply assume one noise sample per observation, but we can trivially extend it to any integer $K>0$ and in fact asymptotically the estimator gets better performance as we increase K.
Once we’ve estimated our density ratio we can easily recover our normalised probability model of the underlying distribution by adding the log probability density of the noise function and taking the exponential.
This estimator is consistent, efficient and asymptotically normal. In [1] they also showed it working empirically in a range of different settings.
How does it compare to other estimators of unnormalised parameterised probability density models?
NCE is not the only method we can use to solve the problem of estimating an unnormalised parameterised probability model. As we mentioned NCE belongs to a family of methods named Energy Based Models (EBM’s) which all aim to solve this very problem of estimating an unnormalised probability model. Let’s very briefly mention some of the alternatives from this family of methods, please do check out the references in this sub-section if you would like to learn more. We will talk about the methods as they appeared in their seminal form.
One alternative is called contrastive divergence which estimates an unnormalised parametrised probability model by using a combination of MCMC and the KL divergence. Contrastive Divergence was originally introduced with Boltzmann machines in mind [9], MCMC is used to generate samples of the activations of the Boltzmann machine and then the KL divergence measures the difference between the distribution of the activations given by the real data and the simulated activations. We then aim to minimise the KL divergence.
Score matching [11] models a parameterised probability model without the computation of the normalising term by estimating the gradient of the log density which it calls the score function. It does this by minimising the expected square distance between the score function and the score function of the observed data. However, obtaining the score function of the observed data requires estimating a non-parametric model from the data. They magically avoid doing this by deriving an alternative form of the objective function, through partial integration, leaving only the computation of the score function and it’s derivative.
Importance sampling [10], which has been around for quite a while uses a weighted version of MCMC to focus on parts of the distribution that are ‘more important’ and in the process self-normalises. Which makes it better than regular MCMC because you can use it on unnormalised probability models and it should be more efficient and have lower variance.
[1] contains a simple comparison between NCE, contrastive divergence, importance sampling and score matching. In their experimental setting they found contrastive divergence got the best performance, closely followed by NCE. They also measured computation time and found NCE to be the best in terms of error versus computation time. This by no means crowns NCE as the best estimator but is a good suggestion as to it’s utility, so is the countless ways it’s been used with high efficacy on a multitude of real-world problems.
Building on Binary-NCE (Ranking-NCE and Info-NCE)
Taking inspiration from Binary-NCE a number of other estimators have been devised. One such estimator is Ranking-NCE [2]. This estimator has two important elements.
The first is that the estimator assumes that we are trying to model a conditional distribution, for example $p(y|x)$. By making this assumption our normalising constant is different for each value of the random variable we are conditioning on, ie. Our normalising term is now some $Z(x;\theta)$ and we have one for each possible value of x. This loosens the constraints on our estimator as we don’t require our optimal parameters, $\theta^{*}$, to satisfy $\log Z(x;\theta^{*}) = c$ for some $c$ for all possible values of $x$. This means we can apply our model to problems where the number of possible values of $x$ is much larger than the number of parameters in our model. For further details on this please refer to [2], section 2.
The second is that it has an objective that given an observed sample $x$, and an integer $K>1$ samples from the noise distirbution, the objective ranks the samples in order of how likely they were to have come from the model versus the noise distribution. Again for further details please refer to [2].
Importantly this version of the estimator can be applied to more complex problems and empirically has been shown to achieve better performance.
Now what we’ve been waiting for … how can we use NCE for representation learning? This is where Info(rmation) NCE comes in. It essentially is Ranking-NCE but we chose our conditional distribution and noise distribution in a specific way.
We consider a conditional probability of the form p(y|x) where $y \in \mathbb{R}^{d_{y}}$, $x \in \mathbb{R}^{d_{x}}$, $d_{y} < d_{x}$. Where $x$ is some data and $y$ is the low-dimensional representation we would like to learn for $x$. We then choose our noise distribution, $p_{n}$, to be the marginal distribution of our representation $y$, $p_{y}$. So our density ratio becomes.
$
\begin{aligned}
\frac{p_{m}(y|x; \theta)}{p_{y}(y)}
\end{aligned}
$
This is now a measure of how likely a given $y$ is to have come from the conditional distribution we are trying to model, ie. how likely is this representation to have been obtained from $x$, versus being some randomly sampled representation.
A key thing to notice is that we are unlikely to have an analytical form of the $log$ of the marginal distribution of $y$. In fact, this doesn’t matter as we aren’t actually interested in modelling the conditional distribution in this case. What we are interested in is the fact that by employing a Ranking-NCE style estimator and modelling the above density ratio we maximise a lower bound on the mutual information between $Y$ and $X$, $I(Y;X)$. A proof for this along with the actual objective function can be found in [3].
This is quite an amazing result! We solve a proxy problem of a proxy problem and we get an estimator with great theoretical guarantees that is computationally efficient that maximises a mutual information which allows us to, in an unsupervised manner, learn general representations for data. So we avoid our problems twice! I appreciate that above were two big jumps with not much detail but I hope it gives a sense as to the link between NCE in it’s basic form and representation learning. More specifically, NCE is known as a self-supervised learning method which simply means an unsupervised method which uses supervised methods but generates its own teaching signal. Even more specifically, NCE is a contrastive method which gets its name from the fact that it contrasts samples against each other in order to learn. The other popular category of self-supervised learning methods are called generative models, you may have heard of these!
My Research
Now we know a little bit about NCE and how we can use it to do representation learning, what am I researching?
Info-NCE has been applied with great success in many self-supervised representation learning techniques, a good one to check out is [4]. Contrastive self-supervised learning techniques have been shown to outperform supervised learning in many areas. They also solve some of the key challenges that face generative representation learning techniques in more challenging domains than language such as images and video. This review [5] is a good starting point for learning more about what contrastive learning and generative learning are and some of their differences.
However, there are still lots of problem areas where applying NCE, without very fancy neural network architectures and techniques, doesn’t do so well or outright fails. Moreover, many of these techniques introduce extra requirements on memory, compute or both. Additionally, they can often be highly complex and their ablation studies are poor.
Currently, I’m looking at applying new kinds of density ratio estimation methods to representation learning, in a similar way to info-NCE. These new density ratio estimation techniques when applied in the correct way will hopefully lead to representation learning techniques that are more capable in problem areas such as multi-modal learning [6], multi-task learning [7] and continual learning [8].
Currently, of most interest to me is multi-modal learning. This is concerned with learning a joint representation over data comprised of more than one modality, eg. text and images. By being able to learn representations on data consisting of multiple modalities it’s possible to learn higher quality representations (more information) and makes us capable of solving more complex tasks that require working over multiple modalities, eg. most robotics tasks. However, multi-modal learning has a unique set of difficult challenges that make naively using representation learning techniques on it challenging. One of the key challenges is balancing a trade-off between learning to construct representations that exploit the synergies between the modalities and not allowing the quality of the representations to be degraded by the varying quality and bias of each of the modalities. We hope to solve this problem in an elegant and simple manner using density ratio estimation techniques to create a novel info-NCE style estimator.
Hope you enjoyed! If you would like to reach me or read some of my other blogposts (I have some more in-depth ones about NCE coming out soon) then checkout my website at /phd.h-0-0.com.
References
[1] :
Gutmann, M. and Hyvärinen, A., 2010, March. Noise-contrastive estimation: A new estimation principle for unnormalized statistical models. In Proceedings of the thirteenth international conference on artificial intelligence and statistics (pp. 297-304). JMLR Workshop and Conference Proceedings.
[2] :
Ma, Z. and Collins, M., 2018. Noise contrastive estimation and negative sampling for conditional models: Consistency and statistical efficiency. arXiv preprint arXiv:1809.01812.
[3] :
Oord, A.V.D., Li, Y. and Vinyals, O., 2018. Representation learning with contrastive predictive coding. arXiv preprint arXiv:1807.03748.
[4] :
Chen, T., Kornblith, S., Norouzi, M. and Hinton, G., 2020, November. A simple framework for contrastive learning of visual representations. In International conference on machine learning (pp. 1597-1607). PMLR.
[5] :
Liu, X., Zhang, F., Hou, Z., Mian, L., Wang, Z., Zhang, J. and Tang, J., 2021. Self-supervised learning: Generative or contrastive. IEEE transactions on knowledge and data engineering, 35(1), pp.857-876.
[6] :
Baltrušaitis, T., Ahuja, C. and Morency, L.P., 2018. Multimodal machine learning: A survey and taxonomy. IEEE transactions on pattern analysis and machine intelligence, 41(2), pp.423-443.
[7] :
Zhang, Y. and Yang, Q., 2021. A survey on multi-task learning. IEEE Transactions on Knowledge and Data Engineering, 34(12), pp.5586-5609.
[8] :
Wang, L., Zhang, X., Su, H. and Zhu, J., 2024. A comprehensive survey of continual learning: Theory, method and application. IEEE Transactions on Pattern Analysis and Machine Intelligence.
[9] :
Carreira-Perpinan, M.A. and Hinton, G., 2005, January. On contrastive divergence learning. In International workshop on artificial intelligence and statistics (pp. 33-40). PMLR.
[10] :
Kloek, T. and Van Dijk, H.K., 1978. Bayesian estimates of equation system parameters: an application of integration by Monte Carlo. Econometrica: Journal of the Econometric Society, pp.1-19.
[11] :
Hyvärinen, A. and Dayan, P., 2005. Estimation of non-normalized statistical models by score matching. Journal of Machine Learning Research, 6(4).